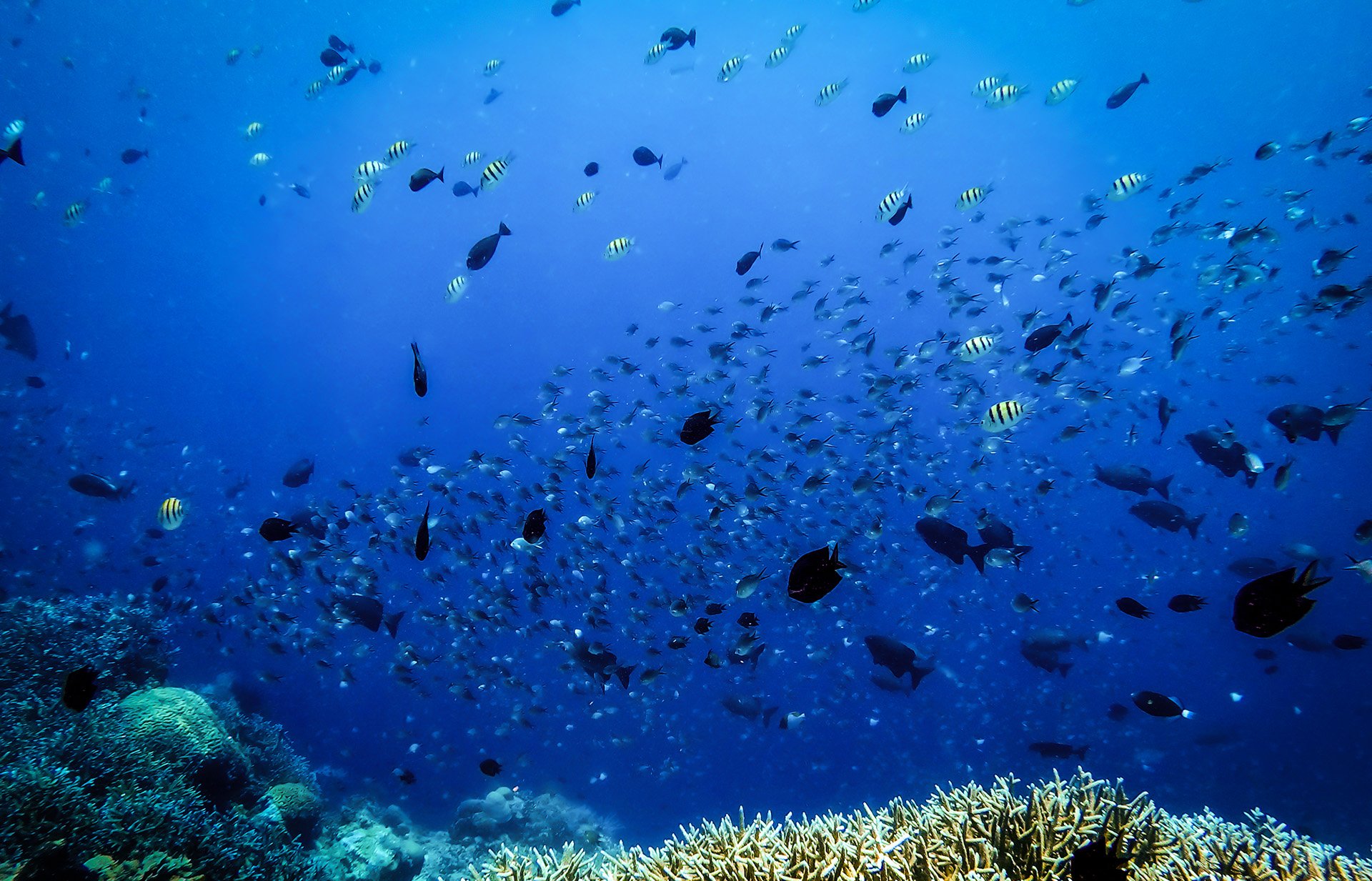
COMPUTATIONAL ECOLOGY AND BEHAVIOR
Computational Ecology and Behavior
Welcome to the Hein Lab based in the Computational Biology department @ Cornell University. We work on a range of fundamental problems in theoretical and computational biology. We are particularly interested in the neural and computational mechanisms by which organisms control their behavior, and in how behavior scales up to influence processes at the scale of biological collectives and ecological systems.
Recent Press
Recent Publications
Wild animals suppress the spread of socially-transmitted misinformation. PNAS
Demystifying image-based machine learning. Front. Marine Sci.
Merging computational fluid dynamics and machine learning to reveal animal migration strategies. Methods Ecol. Evol.
Fast behavioral feedbacks make ecosystems sensitive to pace and not just magnitude of environmental change. PNAS
An algorithmic approach to natural behavior. Current Biology
Recent Funding
NSF: Rules of Life Emerging Networks: The biology of “multivalent” interactions
NOAA: Machine vision tools for underwater imagery
NSF: Flexibility and robustness of attack and evasion behavior
Join the lab
We are always looking for dynamic new scientists, mathematicians, and engineers to join the lab. Interested graduate, postdoctoral, or undergraduate researchers should contact Andrew to inquire about possibilities.